Recently, Dr. Miaohua Mao's research team at the Yantai Institute of Coastal Zone Research, Chinese Academy of Sciences, has developed a fast storm surge prediction method, which improves the quality of typhoon wind field by using a hybrid wind field. Four Machine Learning (ML) models were built to predict storm surges that significantly improve prediction accuracy when combined with Finite Volume Community Ocean Model (FVCOM-ML). The research is published in the Journal of Geophysical Research: Machine Learning and Computation, which is a professional academic journal in the field of earth, space and environmental sciences created by American Geophysical Union (AGU) and maintains a high international academic reputation and peer recognition.
Accurate and timely storm surge prediction provides critical information in coastal zone management and risk reduction strategies. The semi‐enclosed Bohai Sea in the Northwest Pacific that used to be less prone to typhoon disasters, has been witnessing a paradigm shift in typhoon activities recently. Therefore, accurately predicting storm surges is crucial to protecting lives and property in coastal areas. In this study, a hybrid wind field is constructed using the reanalysis wind field and the Holland model, and four ML models are developed by compensating for missing observations through numerical simulations of storm surge performed by the Advanced Circulation Model (Figure 1). The accuracy of storm surge prediction is improved, and the uncertainty is reduced by forecasting the storm surge with lead time of 6, 12 and 18 h (Figure 2 and 3) by combining with FVCOM (FVCOM-ML) (Figure 4).
The difference in forecast accuracy of the four ML models for single-site and single-step storm surge is not significant, while it decreases with the increase of the forecast lead time. The prediction accuracy of the integrated model for single-site and multi-step is more than 1/3 higher than that of the single model. The forecasting ability of the integrated model for multi-site storm surge is significantly better than that of single models, especially for multi-site-multi-step. Combining ML and numerical models, FVCOM-CNN-LSTM and FVCOM-ConvLSTM show high prediction ability. Unlike ML, which only follows the objective function and does not follow the physical principles, FVCOM‐ML simulates the residuals that effectively reduce the uncertainty inherent in traditional methods. The prediction accuracy of the ML model in Bohai and Laizhou Bays is higher than that of Liaodong Bay for the storm surges. Compared with single models, the integrated models can improve the accuracy of storm surge prediction in the Bohai Sea by 18%. The cost of storm surge prediction can be reduced by the ML technique, which makes the ML models expected to be a rapid response prediction system for storm surge in the future.
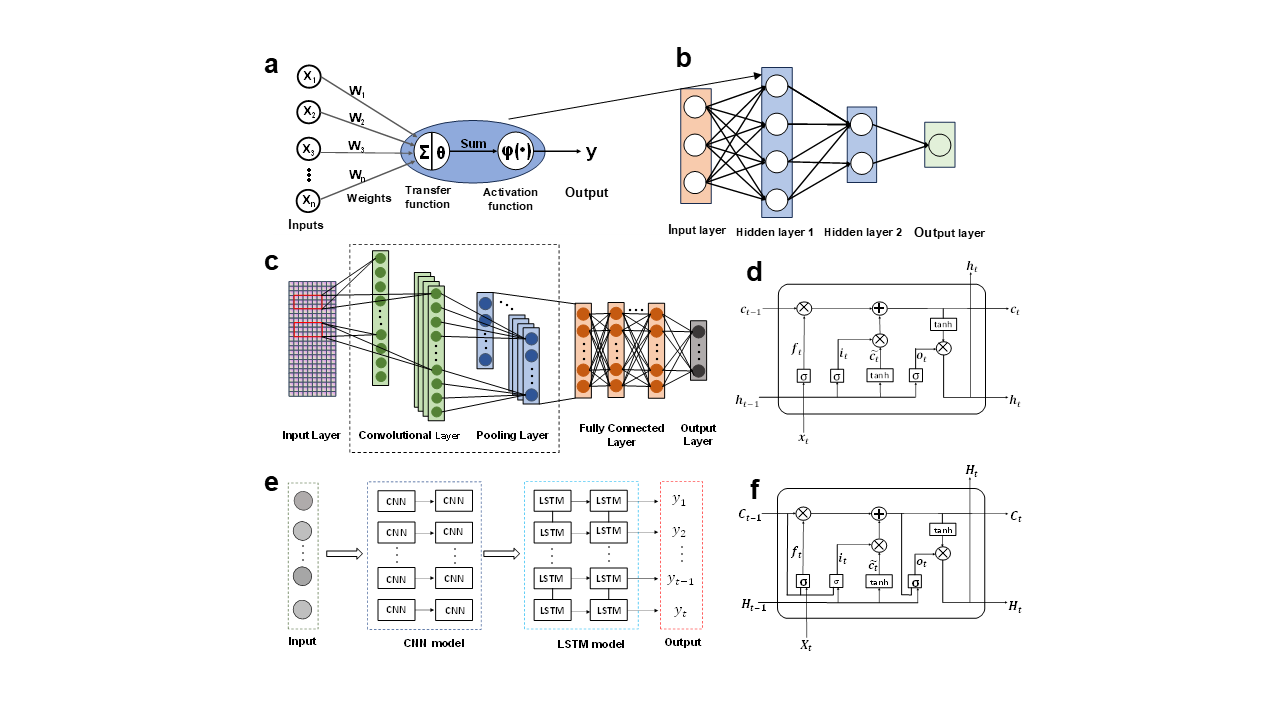
Figure 1. Schematic diagram of the ML models
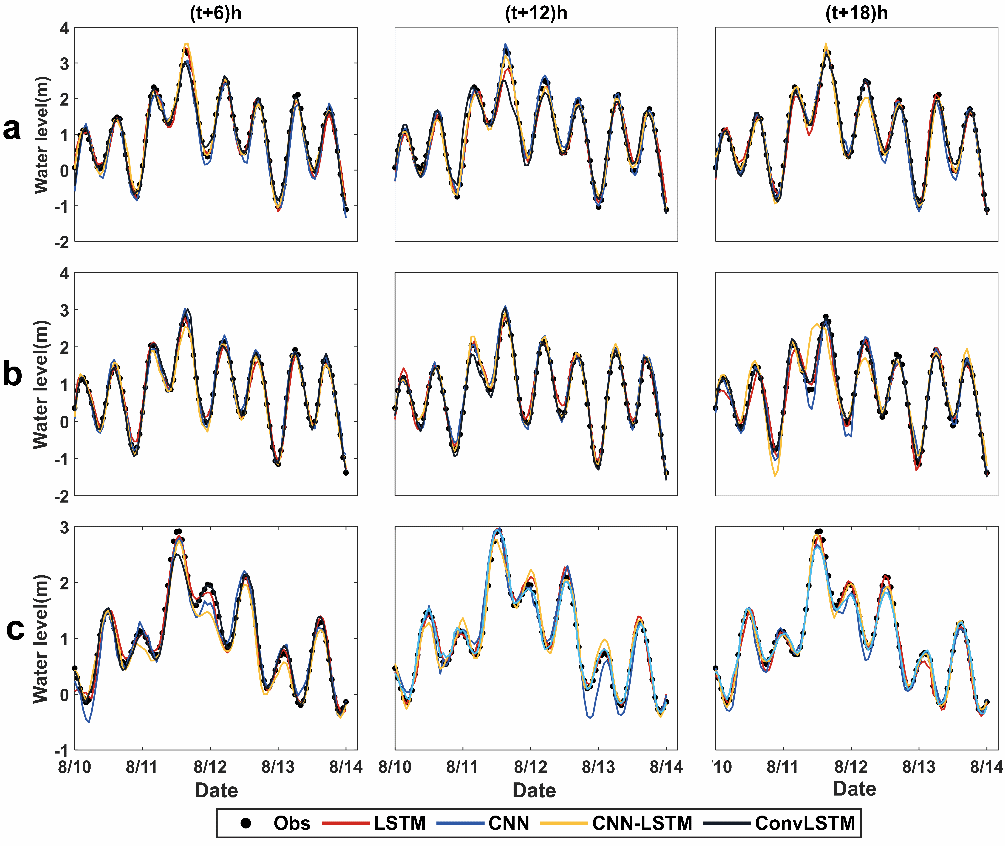
Figure 2. ML models prediction of storm surge versus observed data
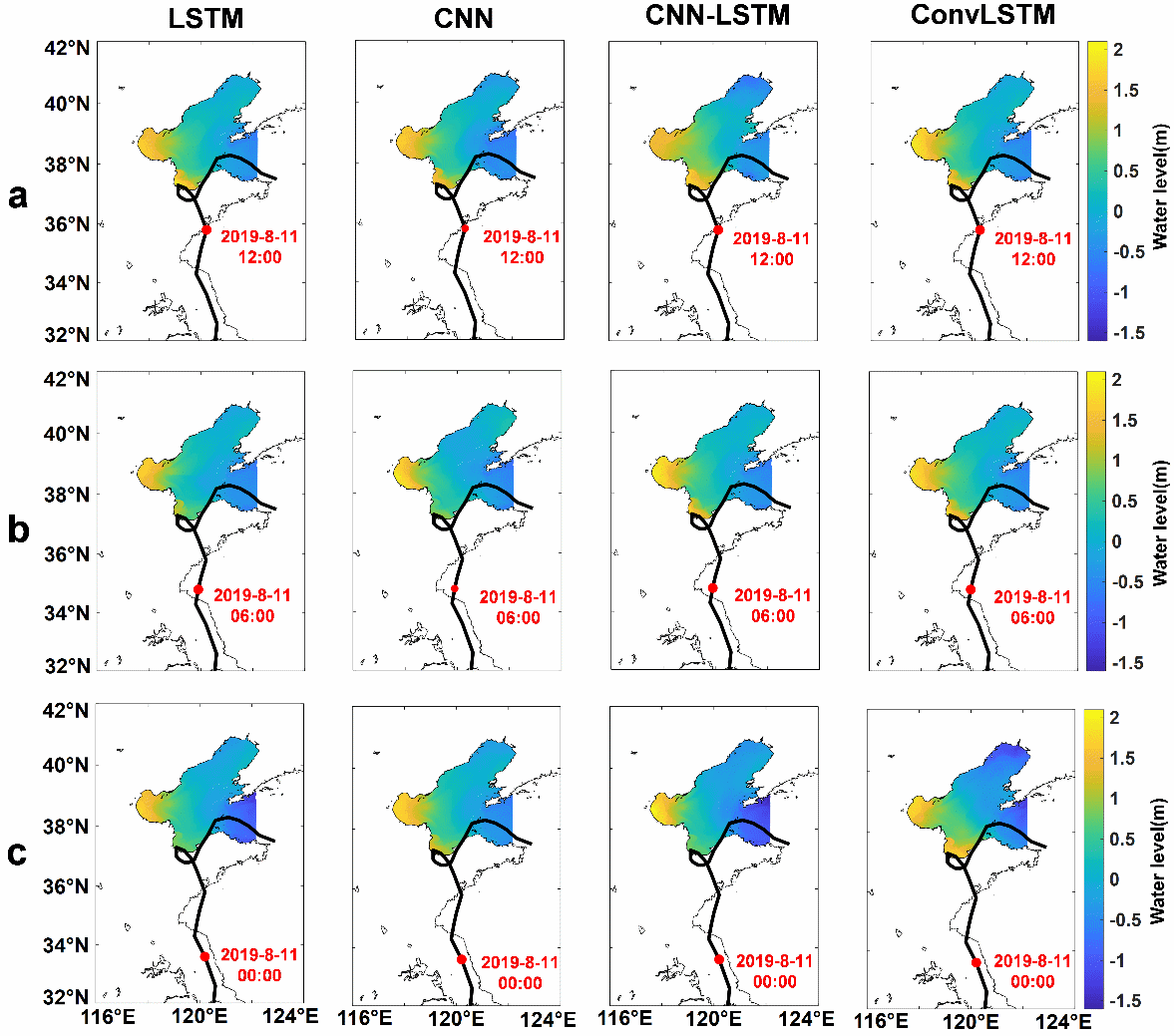
Figure 3. The ML models predict storm surge of ‘Lekima 1909’ with lead time of 6, 12 and 18h.
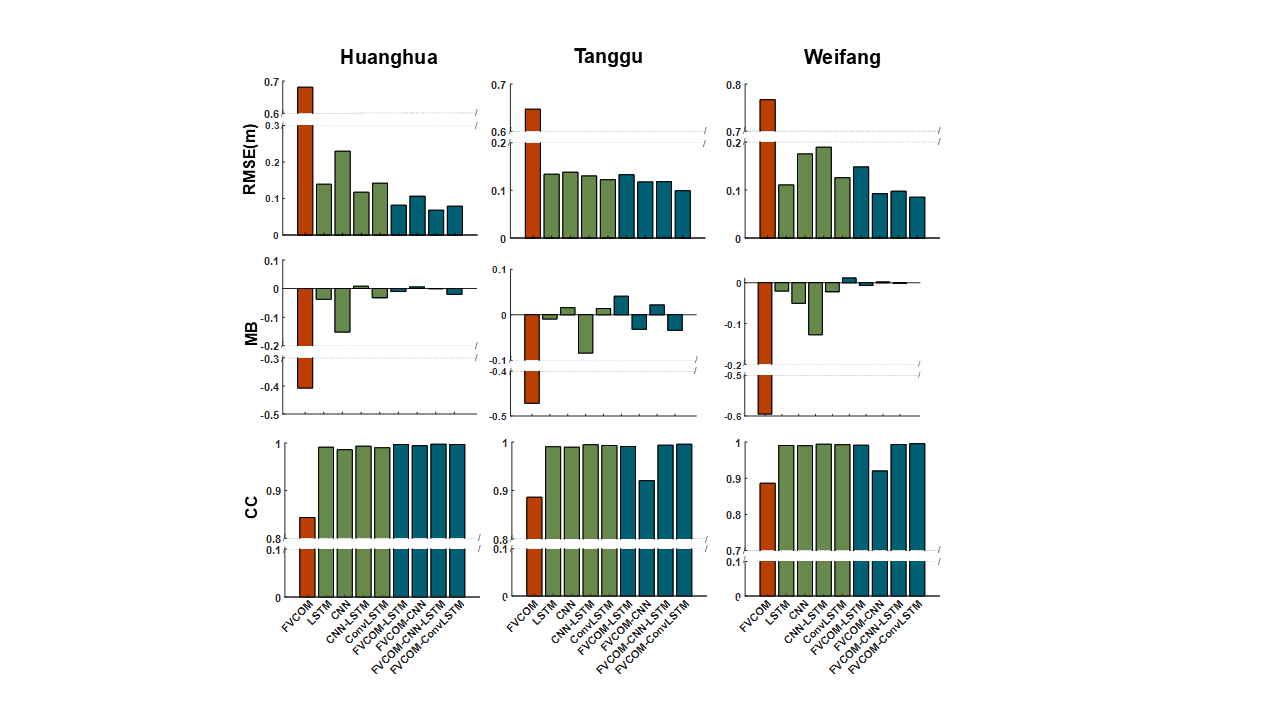
Figure 4. Comparison of FVCOM, ML and FVCOM-ML results for storm surge prediction
The first author of this paper is Changyu Su, a PhD student at the Yantai Institute of Coastal Zone Research, Chinese Academy of Sciences, co-authored by Prof. Miaohua Mao (advisor of Su), Bishnupriya Sahoo (corresponding author) and Dr. Meng Xia in the University of Maryland. This work is supported by the National Natural Science Foundation of China (42050410325), the CAS President's International Fellowship (2020FYM0003), Yantai City Double Hundred Plan Elite Program (E039031101) and Key R and D program of Shandong Province, China (2022CXPT019).
Paper Information:
Su, C., Sahoo, B*., Mao, M., & Xia, M. (2025). Machine learning techniques for predicting typhoon‐induced storm surge using a hybrid wind field. Journal of Geophysical Research: Machine Learning and Computation, 2, e2024JH000507. https://doi.org/10.1029/2024JH000507
Information on related papers:
Peng, J., Mao, M.*, & Xia, M. (2024). Wave Spectra Analysis on the Spatiotemporal Variability of Sea States under Distinct Typhoon Tracks in a Semienclosed Sea. Journal of Physical Oceanography, 54(3), 783-807. https://doi.org/10.1175/JPO-D-23-0066.1
Peng, J., Mao, M.*, & Xia, M. (2023). Dynamics of wave generation and dissipation processes during cold wave events in the Bohai Sea. Estuarine, Coastal and Shelf Science, 280, 108161. https://doi.org/10.1016/j.ecss.2022.108161
Nguyen, Q. T., Mao, M.*, & Xia, M. (2023). Numerical Modeling of Nearshore Wave Transformation and Breaking Processes in the Yellow River Delta with FUNWAVE-TVD Wave Model. Journal of Marine Science and Engineering, 11(7), 1380. https:// doi.org/10.3390/jmse11071380